DMDW Unit-1 Notes
1. Design guidelines for data warehouse implementation
Implementation Guidelines
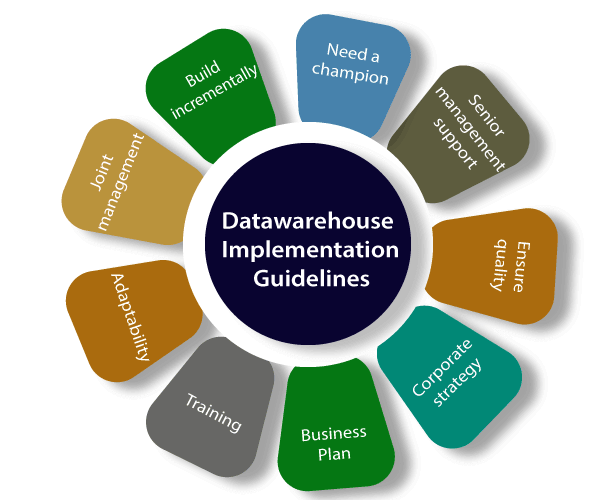
1. Build incrementally: Data warehouses must be built incrementally. Generally, it is recommended that a data marts may be created with one particular project in mind, and once it is implemented, several other sections of the enterprise may also want to implement similar systems. An enterprise data warehouses can then be implemented in an iterative manner allowing all data marts to extract information from the data warehouse.
2. Need a champion: A data warehouses project must have a champion who is active to carry out considerable researches into expected price and benefit of the project. Data warehousing projects requires inputs from many units in an enterprise and therefore needs to be driven by someone who is needed for interacting with people in the enterprises and can actively persuade colleagues.
3. Senior management support: A data warehouses project must be fully supported by senior management. Given the resource-intensive feature of such project and the time they can take to implement, a warehouse project signal for a sustained commitment from senior management.
4. Ensure quality: The only record that has been cleaned and is of a quality that is implicit by the organizations should be loaded in the data warehouses.
5. Corporate strategy: A data warehouse project must be suitable for corporate strategies and business goals. The purpose of the project must be defined before the beginning of the projects.
6. Business plan: The financial costs (hardware, software, and peopleware), expected advantage, and a project plan for a data warehouses project must be clearly outlined and understood by all stakeholders. Without such understanding, rumors about expenditure and benefits can become the only sources of data, subversion the projects.
7. Training: Data warehouses projects must not overlook data warehouses training requirements. For a data warehouses project to be successful, the customers must be trained to use the warehouses and to understand its capabilities.
8. Adaptability: The project should build in flexibility so that changes may be made to the data warehouses if and when required. Like any system, a data warehouse will require to change, as the needs of an enterprise change.
9. Joint management: The project must be handled by both IT and business professionals in the enterprise. To ensure that proper communication with the stakeholder and which the project is the target for assisting the enterprise's business, the business professional must be involved in the project along with technical professionals.
Data Warehouse Design
A data warehouse is a single data repository where a record from multiple data sources is integrated for online business analytical processing (OLAP). This implies a data warehouse needs to meet the requirements from all the business stages within the entire organization. Thus, data warehouse design is a hugely complex, lengthy, and hence error-prone process. Furthermore, business analytical functions change over time, which results in changes in the requirements for the systems. Therefore, data warehouse and OLAP systems are dynamic, and the design process is continuous.
There are two approaches
- "top-down" approach
- "bottom-up" approach
Top-down Design Approach
In the "Top-Down" design approach, a data warehouse is described as a subject-oriented, time-variant, non-volatile and integrated data repository for the entire enterprise data from different sources are validated, reformatted and saved in a normalized (up to 3NF) database as the data warehouse. The data warehouse stores "atomic" information, the data at the lowest level of granularity, from where dimensional data marts can be built by selecting the data required for specific business subjects or particular departments. An approach is a data-driven approach as the information is gathered and integrated first and then business requirements by subjects for building data marts are formulated. The advantage of this method is which it supports a single integrated data source. Thus data marts built from it will have consistency when they overlap.
Advantages of top-down design
Data Marts are loaded from the data warehouses.
Developing new data mart from the data warehouse is very easy.
Disadvantages of top-down design
This technique is inflexible to changing departmental needs.
The cost of implementing the project is high.
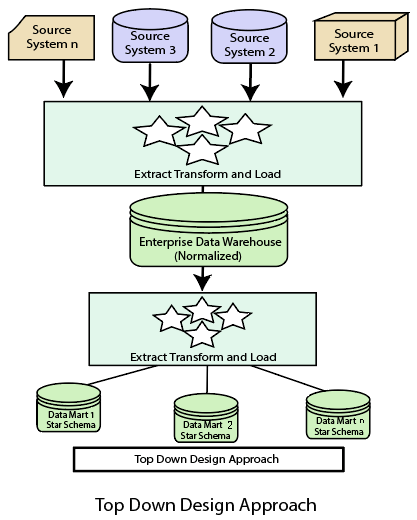
Bottom-Up Design Approach
In the "Bottom-Up" approach, a data warehouse is described as "a copy of transaction data specifical architecture for query and analysis," term the star schema. In this approach, a data mart is created first to necessary reporting and analytical capabilities for particular business processes (or subjects). Thus it is needed to be a business-driven approach in contrast to Inmon's data-driven approach.
Data marts include the lowest grain data and, if needed, aggregated data too. Instead of a normalized database for the data warehouse, a denormalized dimensional database is adapted to meet the data delivery requirements of data warehouses. Using this method, to use the set of data marts as the enterprise data warehouse, data marts should be built with conformed dimensions in mind, defining that ordinary objects are represented the same in different data marts. The conformed dimensions connected the data marts to form a data warehouse, which is generally called a virtual data warehouse.
The advantage of the "bottom-up" design approach is that it has quick ROI, as developing a data mart, a data warehouse for a single subject, takes far less time and effort than developing an enterprise-wide data warehouse. Also, the risk of failure is even less. This method is inherently incremental. This method allows the project team to learn and grow.
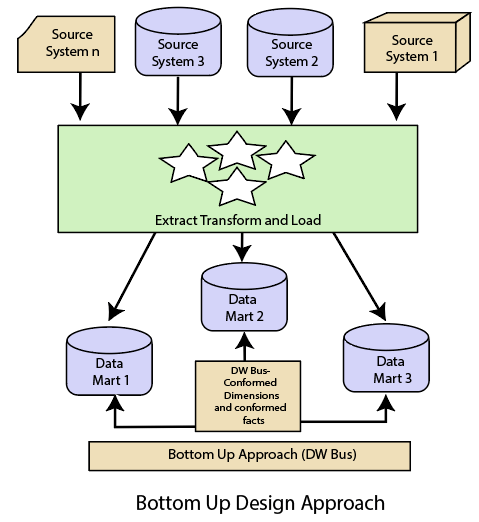
Advantages of bottom-up design
Documents can be generated quickly.
The data warehouse can be extended to accommodate new business units.
It is just developing new data marts and then integrating with other data marts.
Disadvantages of bottom-up design
the locations of the data warehouse and the data marts are reversed in the bottom-up approach design.
Differentiate between Top-Down Design Approach and Bottom-Up Design Approach
Top-Down Design Approach | Bottom-Up Design Approach |
---|---|
Breaks the vast problem into smaller subproblems. | Solves the essential low-level problem and integrates them into a higher one. |
Inherently architected- not a union of several data marts. | Inherently incremental; can schedule essential data marts first. |
Single, central storage of information about the content. | Departmental information stored. |
Centralized rules and control. | Departmental rules and control. |
It includes redundant information. | Redundancy can be removed. |
It may see quick results if implemented with repetitions. | Less risk of failure, favorable return on investment, and proof of techniques. |
2. MULTIDIMENSIONAL MODALS
The multi-Dimensional Data Model is a method which is used for ordering data in the database along with good arrangement and assembling of the contents in the database.
The Multi Dimensional Data Model allows customers to interrogate analytical questions associated with market or business trends, unlike relational databases which allow customers to access data in the form of queries. They allow users to rapidly receive answers to the requests which they made by creating and examining the data comparatively fast.
OLAP (online analytical processing) and data warehousing uses multi dimensional databases. It is used to show multiple dimensions of the data to users.
It represents data in the form of data cubes. Data cubes allow to model and view the data from many dimensions and perspectives. It is defined by dimensions and facts and is represented by a fact table. Facts are numerical measures and fact tables contain measures of the related dimensional tables or names of the facts.
D
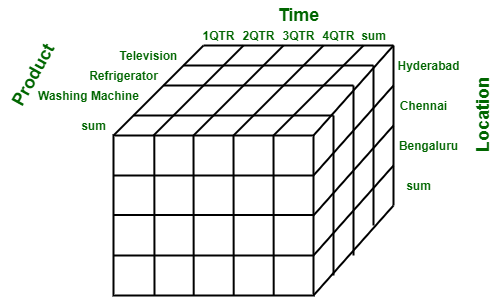
Multidimensional Data Representation
The following stages should be followed by every project for building a Multi Dimensional Data Model :
Stage 1 : Assembling data from the client
Stage 2 : Grouping different segments of the system .
Stage 3 : Noticing the different proportions
Stage 4 : Preparing the actual-time factors and their respective qualities
Stage 5 : Finding the actuality of factors which are listed previously and their qualities
Stage 6 : Building the Schema to place the data, with respect to the information collected from the steps above
Disadvantages of Multi Dimensional Data Model
The following are the disadvantages of a Multi Dimensional Data Model :
- The multi-dimensional Data Model is slightly complicated in nature and it requires professionals to recognize and examine the data in the database.
- The path to achieving the end product is complicated most of the time.
Advantages of Multi Dimensional Data Model
The following are the advantages of a multi-dimensional data model :
- A multi-dimensional data model is easy to handle.
- It is easy to maintain.
- Its performance is better than that of normal databases (e.g. relational databases
Comments
Post a Comment